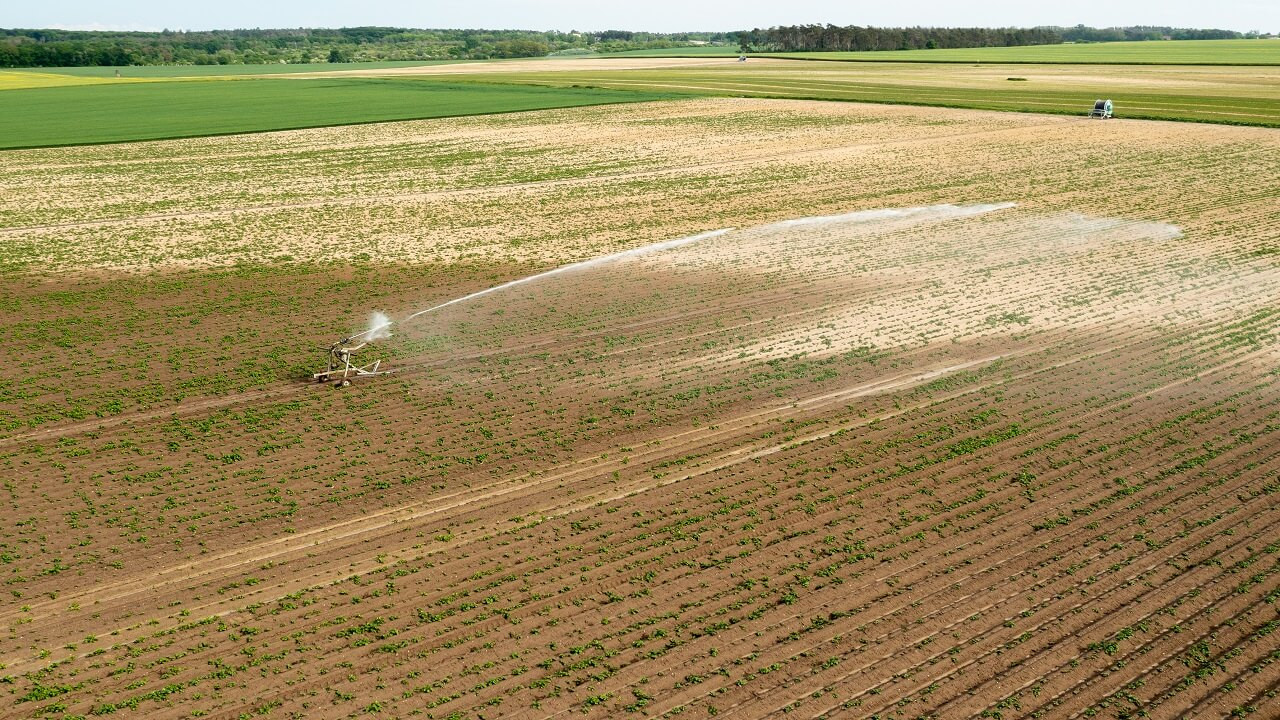
Harnessing The Power Of Technology: Crop Yield Prediction In Agriculture
August 9, 2023, 7:30 am
Crop yield is the measurement of the quantity of agricultural crops collected per unit of land.
It is usually applied to grains and cereal crops and is measured in tons or pounds per acre. Based on the weight of the harvested crop, farmers then calculate yields for the entire field, extrapolating the resulting products to the whole farm.
The forecasting of yield is an example of how spatial data science is being applied in today's world. Satellite imagery that helps generate forecasting algorithms is also used by companies, government agencies, and academic institutions to make data-driven decisions.
The algorithms, in turn, help assess and determine the soil's capacity for moisture and nutrient content. They are also helpful in identifying factors that limit crop development.
In addition to providing food, agriculture also plays an essential role in the economy and employment, as about 30% of the world's labor force is employed in agriculture. Food and crop production affect population size. The population can only increase with enough food. Better agriculture can lead to population growth. In England, new farming methods caused the people to almost triple in just a century.
The Haber-Bosch fertilization process, invented in the 1900s, significantly increased the world's population This growth can be attributed to advancements in crop production, both in the UK and globally. Given the crucial role of arable farming, predicting crop yields has been a longstanding practice in agriculture.
How Crop Yield Forecasting Works
Various data sources given different weights based on the prevailing circumstances should be used to determine yield projections for the current cropping season in other regions. The data scientists use machine learning and various data sources to update the yield forecast estimates whenever a major yield component is affected.
Accurate preseason data is crucial for predicting crop yield. This data can be obtained before planting and sets the foundation for the initial yield predictions. It's essential to consider location, past crops, soil tests, and weather data from the last ten years to make smart choices for planting or seeding.
The data that affects crop yield during a growing season is specific to each plant. It includes information such as the amount of water present during critical growth stages or the number of hours the crops are exposed to certain temperatures during crucial periods that determine yield. This information is essential for predicting the yield of the five main crops: corn, canola, lentils, soybeans, and wheat.
Researchers use high-resolution satellite imagery to quickly and accurately determine NDVI crop health values on fields. It helps detect crop issues on the scale of an entire region that may impact yield. By analyzing regional trends, they can decide if areas meet forecasted expectations or if environmental factors affect the predictions.
Technologies in Yield Estimation
In recent years, machine learning (ML) has become a powerful tool for yield prediction. This branch of artificial intelligence allows computers to learn from data without being programmed. It is an excellent tool for predicting yield as it can detect patterns and relationships within large data sets and use this information to make them accurate. Different types of machine learning algorithms can be used for crop yield forecast.
Regression algorithms use input data, including weather data, soil data and management practices, to predict yield. They are simple to understand and easy to implement.
Decision tree algorithms utilize a tree structure to model decisions and their potential outcomes. The algorithm begins by deciding based on the most significant input factor and then makes further decisions based on subsequent input factors.
Regarding machine learning, artificial neural networks are a more advanced algorithm miming how the human brain works. They're great at predicting crop yields because they can handle big data sets and spot complicated patterns and connections.
After a machine learning algorithm has been trained, it can be utilized to forecast yields in new plots. For the algorithm to make predictions, it needs to be provided with relevant data, such as weather conditions and soil characteristics.
EOSDA Crop Yield Prediction
Effective food management practices are critical to global food security yield forecasting helps farmers grow crops more sustainably.
EOS Data Analytics utilizes AI technologies to provide satellite data analytics to over 20 different industries. The company has also developed effective crop yield estimation using remote sensing and machine learning technologies.
The yield forecasting solution created by EOSDA is designed to assist farmers, agribusinesses, food security companies and other decision-makers in sustainable and profitable crop production. This solution employs cutting-edge technology, including machine learning and geospatial analytics, to deliver crucial data.
EOSDA yield forecasts can be 95% accurate (depending on the quality of statistical data). The production takes a week or less, depending on the project's complexity. Yield forecasting up to 3 months in advance is available for more than 100 crop types and is based on the most comprehensive data analysis from more than ten sources.
Share This Article: